Dr Aniruddha Pant, PhD. CEO, AlgoAnalytics, Pune, India
Dr Sudhanshu Patwardhan, MBBS, MS, MBA. Director, Centre for Health Research and Education, Hampshire, UK
Dr Sharda Bapat, MD. CSO, AlgoAnalytics, Pune, India
Tobacco usage in India is one of the largest preventable cause of non-communicable diseases such as oral cancer, lung cancer, obstructive lung disease and cardiovascular disease, to name a few. Consumption of tobacco in smoked forms (e.g., cigarettes, bidis, cigars, hookah) and smokeless forms (e.g., Gutkha, zarda, khaini) kills nearly a million users annually- all entirely preventable premature deaths.
An epidemic with no sign of abating
With nearly 300 million current users of risky tobacco in India, of which 200 million chew smokeless tobacco, the tobacco use epidemic and the resultant deaths from cancers and other preventable diseases are unlikely to abate for the foreseeable future. The effects of smokeless tobacco are most pronounced in the oral cavity, manifesting as submucosal fibrosis, leukoplakia and oral cancers. The incidence of oral cancer in India is among the highest worldwide, comprising nearly half of all oral cancers globally. Already, smokeless tobacco kills more than 350,000 people every year in India. Particularly concerning is the disproportionately higher use of smokeless tobacco among socio-economically disadvantaged groups, women and the rural population. For example, tobacco chewing is common among migrant construction workers, those living in urban slums, truck and metro transport drivers. In the case of pregnant women chewing tobacco, their tobacco use harms them as well as their babies with an increased risk of anaemia, higher rates of stillbirth and lower weight of the newborn.
Catch them early and save millions of lives
The user groups of smokeless tobacco often have a poor understanding of tobacco harms and cessation aids, along with poor access to healthcare. Cessation aids in the form of behavioural support and safer nicotine alternatives to smokeless tobacco are not affordable, accessible or available to the majority of the population.
Screening for pre-malignant and early-stage oral cancers can be done by experts by visual inspection. However, if the person at the point of care (POC) who is screening is not skilled and experienced, then the sensitivity and specificity of the visual diagnosis can be very low. This is generally the case in rural healthcare settings. As a result, tobacco user patients from rural and disadvantaged backgrounds are more likely to present late with stage III or stage IV oral cancer. Currently in India, overall survival following treatment at 5 years for Stage I oral cancer is 100% and decreases to 85% for Stage II. For Stages III and IV, 5-year survival can be a mere 43% and 42% respectively. This is further worsened due to lower affordability and access to chemotherapy and radiotherapy for most of the rural populations.
Early screening of pre-cancerous oral lesions such as leukoplakia and erythroplakia complemented by opportunistic and comprehensive tobacco cessation support has the potential to be a significant and impactful public health intervention. So how do we achieve this breakthrough soon and to scale? Can digital technology and machine learning come to the rescue?
Artificial Intelligence enabled POC screening
Computer vision has a great potential to help the Healthcare industry. All diagnoses which involve visual inspections of photographs or scans of body parts (Radiology, Ophthalmology, Skin) and tissue samples (histopathology) are considered to be ripe application areas.
Identifying pre-malignant lesions is ultimately a pattern detection and pattern localization problem. This is where a well-designed, robustly tested and scientifically validated Artificial Intelligence (AI) algorithm can be a game-changer. If experts such as oral pathologists, dentists and cancer surgeons come together to collect, label and correctly classify hundreds of unique images of oral lesions using standardised protocols, these can be used to train the AI algorithm to do the job in an automated fashion for future images. Through the process of iterative testing and continual machine learning, created by machine learning scientists and guided by medical experts, a reliable and field-worthy screening tool is possible. Ultimately, this can be taken to a mobile device that can take images of the oral cavity at the POC as well as spit out (pun intended) a “red flag” for an expert evaluation if a pre-cancerous lesion is suspected. Such a patient, if a tobacco user, will also be referred to the tobacco control centre. The team led by one of the authors of this article has done multiple successful commercial deployments of machine learning as applied to Radiology imaging. More than 25 different algorithms analysing X-rays, CT scans and MRI’s to create pathology detection and localization algorithms have been developed. Using this approach we have been able to provide AI-based tools to Radiologists which allow them to improve accuracy, efficiency manifold and avoid burnout. Automatic triaging tools for pandemics like Tuberculosis and community-acquired pneumonia have been created and they are being used to mark potentially at-risk individuals who can be further confirmed by Radiologist or a pathology test.
Machine learning enabled by Human expertise
This experience of last 5–6 years in medical image analysis has taught a lot of lessons to us and we believe these lessons will be very valuable in creating a robust tool that can perform in the real world. Such a tool can be used for social and public health impact rather than building an academic algorithm which works only in laboratory but sees substantial performance deterioration when deployed on data from wild.
For building robust tools one must attack the problem keeping in mind various important factors which are very specific to medical imaging. Some of the critical aspects that we have converged on are given below:
1. Collecting data from various sources and various demographics.
2. Collecting data from various camera devices or specifying a specific device for collecting data.
3. Use manifold image augmentation methods to create robust training dataset.
4. Build algorithms which will detect distribution shift in the field and alert if the output of the algorithm is likely to be wrong and provide appropriate confidence interval around it.
5. Create models which are accurate and robust, but still have a small footprint so that a commonly used mobile device can run these in practice.
6. Provide learning loop which allows us to fine-tune and update models as more and more data becomes available.
7. Do all of this in close collaboration with relevant medical experts.
Pictures below show some samples that could be collected and annotated by an expert in terms of the affected area. These will form a basis to build an AI detection and pathology localization algorithm.
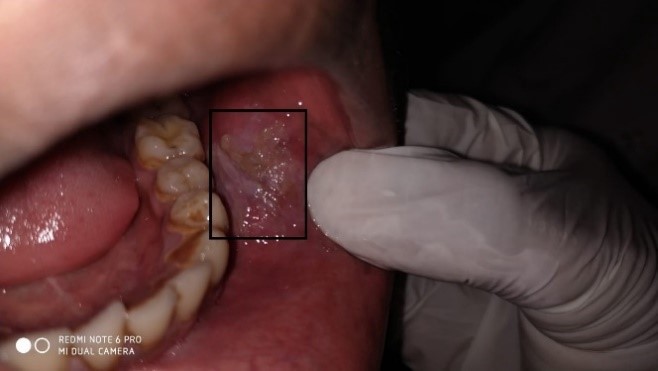
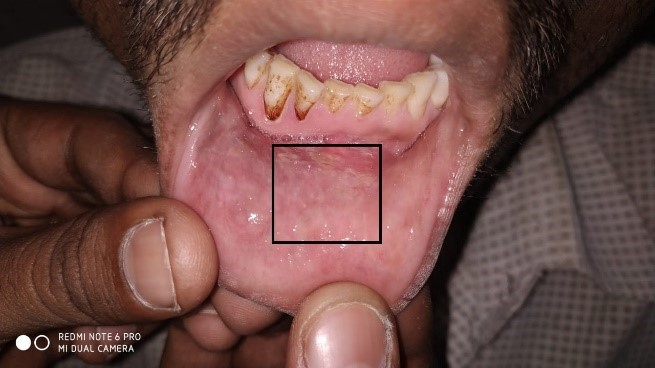
Edge Computing for scalability
Our proposed approach for screening oral cancers at the POC, enabled by edge computing, is unique for many reasons:
1. A massive training dataset is required for achieving a functional AI algorithm with high sensitivity and specificity on diverse datasets. Till now, the technology to capture and share digital images at a large scale from across clinics in India was either non-existent or cost-prohibitive. It is feasible now.
2. A digitally enabled oral cancer screening targeted at tobacco users in rural populations was not feasible due to technological limitations. For example, mobile devices to capture high-resolution digital images at the point of care also needed adequate computational power to run the screening algorithm in a standalone/offline mode. Today’s smartphones with 5G connectivity can make it possible.
3. Motivated health workers will be trained and incentivised to use the smartphones for image capture, screening and referral. Our team’s experience in training healthcare professionals will be crucial to understand and manage healthcare workers’ motivation, and enabling effective delivery of the innovation at the POC.
We are putting together a multi-disciplinary team to achieve and realise this innovation. We believe a multi-disciplinary team is absolutely a must for the successful execution of such a project. Dentists, oral pathologists, clinical research organisations, health workers, database designers, software developers and machine learning experts will be working together in the coming years to develop, test and roll out this screening tool, building on our success in Radiology space. It will be a ‘Made in India’ tool with universal application and the potential to optimise the delivery of tobacco cessation in a timely manner to those who need it the most- and prevent large numbers of premature deaths globally.
This post first appeared in Medium